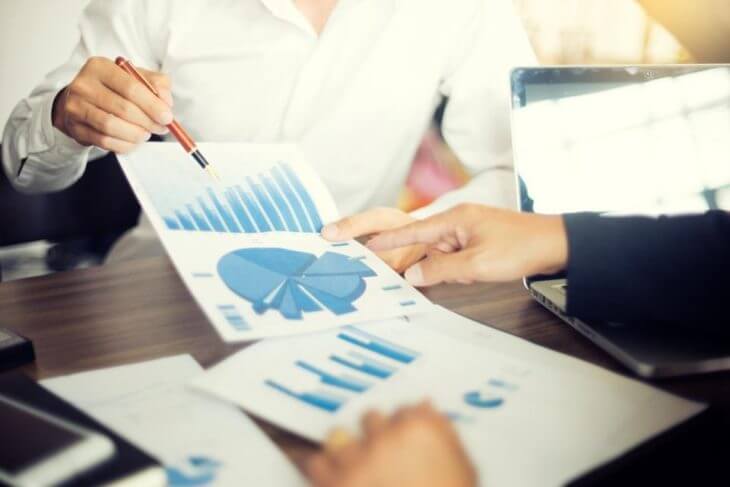
5 practical strategies for being a machine learning marketing expert
The growing prominence of machine learning in marketing is altering the way this industry operates. Without a doubt, this evolution is exciting, since it reveals new possibilities for connecting with customers, using techniques that weren’t available just a couple of years back. But it’s also terrifying, because the race between the data haves and have-nots intensifies, and marketing FOMO becomes relevant. Although it might seem like everyone else has become an AI marketing ninja, the entire industry is actually still in the process of learning. As reported in the study carried out by Salesforce, only 26% of economic leaders are confident their organization is able to develop an AI strategy. It aligns statistically with my very own experience dealing with marketing clients across a variety of industries. In my experience, a lot of companies are at only starting to use data and machine learning within their marketing (but they’re learning rapidly).
The task for marketers at this time is to handle the fact that the appetite for AI-infused marketing solutions is high, and to adapt to these requirements. However, the knowledge of what AI really does is still very low. Unfortunately, this creates an atmosphere where vendors play fast-and-loose using the term “AI” and marketers are at risk of being sold digital snake oil. Being an engineer, this dynamic frustrates me. So, I’ve outlined five practical steps to get smart about marketing with machine learning, in order to help marketers become informed buyers, in case they are evaluating AdTech solutions, interviewing agencies or building their very own in-house data science teams.
1. Define your marketing objectives
Before you begin going through the options of incorporating machine learning into your marketing, you need to specifically define your marketing objectives. Machine learning is a means to an end, that will help you achieve your marketing goals. If you specifically define your goals, you’ll possess a grounded group of criteria for testing and evaluation of machine learning technologies. For instance, in case your goal is awareness, and your objective is to take down cost per acquisition by 15% making it lower than your average customer lifetime value (CLTV), you need to search for solutions which use predictive models to improve conversation rate and/or lessen the cost per impression or click. Alternatively, you may search for solutions which use personalization and smart recommendations to improve the quantity each client spends, therefore growing CLTV. Both strategies use machine learning to enhance your marketing Return on investment.
The important thing to realize is the fact that, despite all the hype, machine learning isn’t about creating a general-purpose AI that replaces your marketing intern. Rather, it’s a group of specialized algorithms made to optimize highly specific tasks using data. Machine learning doesn’t alter the fundamentals of marketing. Like several AdTech/MarTech, you have to assess how likely machine learning technology is to improve your marketing Return on investment after considering the price of the technology itself.
2. Obtain a fundamental knowledge of how machine learning works
Machine learning is a new method of solving issues and it’s essential for all business leaders, especially marketers, to gain fundamental knowledge of how it operates under-the-hood. In case you don’t possess a conceptual mental model for the way machine learning functions, all purported AI solutions may look like magic and you’ll go astray trying to chase shiny objects that do not perform.
So we’ll begin by defining the word correctly: Machine learning is really a subset of the broader field of AI that concentrates on a group of specialized algorithms made to optimize a particular objective (e.g. maximize clicks or identify fraudulent transactions) based to historic and/or real-time data. Except for machine learning, AI includes various fields, but recently, machine learning has dominated so for practical purposes, the term “machine learning” and “artificial intelligence” have grown to be synonymous. Data scientists and engineers like the term “machine learning” while salespeople and futurists usually use the term “AI.”
My two favorite advice for machine learning newbies is to take a look at a group of videos created by Microsoft and Google. They start with a gentle introduction to machine learning before going into details that illustrate how machine learning algorithms work. Another excellent thing to do is to enroll in a web-based course designed for beginners. Udacity launched an Intro to Artificial Intelligence course, lead by two AI heavy-weights, Sebastian Thrun (Udacity’s founder) and Peter Norvig (Director of Research at Google). If you possess the time and inclination, this really is the easiest method to get up-to-speed.
3. Understand what is not considered machine learning
There are a plethora of technologies masquerading as machine learning. Being an informed buyer, you need to look out for the technology buzzwords which are frequently conflated with AI. Here’s a couple of terms you should take into consideration:
Data-Driven Marketing: Using data in marketing doesn’t, by itself, equal machine learning. For instance, you may use first-party customer data to classify readers into certainly one of a number of segments, after which display different happy to users in every segment. This really is data-driven marketing, but it’s not machine learning. Yes, you use data, you will find, you do personalization, however, the rules for segmenting and displaying content are produced by hand with a human. A piece of equipment learning approach, in comparison, might segment users instantly according to historic data utilizing a clustering formula like K-Means, or attempt to predict the information that the user is probably to interact with utilizing a method like Contextual Multi-Arm Bandits.
Big-Data: Many machine learning algorithms, especially neural systems, require huge amounts of data in order to be able to learn. Consequently, big data (i.e. large amounts of data that can’t be stored or processed with a single server) is frequently linked to machine learning. However, big data doesn’t equal machine learning. I’ve been in big data projects in which the finish goal was to provide traditional descriptive analytics (i.e. summary data and dashboards), and I’ve been on machine learning projects which didn’t require big data to create predictions. These two concepts are related, but they are not synonymous.
You should understand machine learning in enough detail so that you can distinguish ML solutions from non-ML solutions. What is really important to remember is that machine learning is the means to an end and not the final goal. Should you stumble upon a good MarTech solution that can help you to achieve your marketing objectives, then it doesn’t really matter if it is using AI or traditional data-driven marketing, as long as it serves the purpose.
4. Make inquiries concerning the technology
When looking for a possible machine learning solution, you shouldn’t be afraid to ask anything you want to know regarding the actual technology. This serves two purposes: 1) it enables you to check out if the vendor is full of hot air or not 2) it’s a terrific way to find out about machine learning free of charge! Here a couple of questions you should ask:
Have you ever published any academic papers? Many early-stage machine learning startups are spun from academia and legitimate data science innovators publish papers on their own work. When the vendor claims to possess a “break-through algorithm” that can serve as the foundation for his or her competitive advantage, ask him where can you read about findings of his study. You will possibly not understand everything, but you’ll figure out if it is real or not.
What are the machine learning algorithms that you use? Any machine learning solution is based on an algorithm, so the easiest method to assess if it is legit or not is to inquire about specific algorithms the organization is applying. You may think this is a really proprietary information, but many people are prepared to share it because ML algorithms are actually a large group of algorithms, and each of them has an enormous amount of variations and optimizations. So by asking what algorithms the merchandise utilizes, you’re really asking about the general method of machine learning they use.
What is the typical amount of a performance lift among your clients? This might be the essential question. Once the AI fairy dust has settled, you need to learn about specific ways this machine learning solution will enhance your marketing Return on investment. Since the concept of machine learning is singularly focused on optimization, a great vendor should be able to give you a clear explanation.
5. Understand in which the creative matches
Machine learning and knowledge can provide many effective results, but it cannot produce compelling marketing creative. Humans possess the unique ability of ideation and creativity. Depending on your objectives (e.g. brand building versus. demand gen), humans might need to be engaged to a great extent. Machine learning vendors are usually technology-focused which is why they frequently downplay the significance of creativeness. But bits and bytes don’t provoke a general change in customer behavior-great creative ideas do. So, it is important for marketers to figure out the way the creative process is going to be influenced by machine learning. Will all creative be optimized for that lowest common denominator? Or is there going to be there be some room for big, bold ideas? How can a creative team use the information and learnings from the data? Each one of these questions will have to be addressed by the marketer evaluating machine learning solutions.
Conclusion
It’s not necessary to become a data researcher to use machine learning and understanding how to use it to enhance your marketing, but you certainly have to gather some valuable fundamental information. Fortunately, with regards to assessing machine learning solutions, a little understanding goes a long way. By asking lots of questions, investigating the subject of machine learning, and adopting a reasonable amount of skepticism, you’ll become an AI marketing ninja very quickly.